引用本文: | 李小刚,高正,陈佳锋,冯山入,傅修涛,丁振斌.单细胞转录组数据批次效应评测的研究进展[J].生物信息学,2023,21(3):155-160. |
| LI Xiaogang,GAO Zheng,CHEN Jiafeng,FENG Shanru,FU Xiutao,DING Zhenbin.Advancement of research measuring batch effects of single cell RNA transcriptome data[J].Chinese Journal of Bioinformatics,2023,21(3):155-160. |
|
|
|
本文已被:浏览 1009次 下载 957次 |
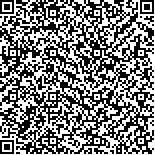 码上扫一扫! |
|
单细胞转录组数据批次效应评测的研究进展 |
李小刚1,2,高正1,2,陈佳锋1,2,冯山入1,2,傅修涛1,2,丁振斌1,2,3
|
(1.复旦大学附属中山医院 肝脏外科与移植外科,复旦大学肝癌研究所,上海 200032; 2.教育部癌变与侵袭原理重点实验室,上海 200032;3.上海市徐汇区中心医院,复旦大学附属中山徐汇医院,上海 200032)
|
|
摘要: |
单细胞转录组测序(Single cell RNA sequencing,ScRNA seq)是一种变革性的生物技术,以前所未有的高分辨率来解析组织复杂性,解决了普通转录组测序(Bulk RNA sequencing)无法回答的问题。但单细胞数据的高通量及复杂性给分析带来极大难度,批次效应(Batch effects,BEs)的处理便是主要挑战之一。批次效应是高通量生物数据分析中的技术性偏倚,其来源及处理具有高复杂性和研究依赖性。根据组织类型、测序技术及实验设计的不同,测序数据需采用不同的评估、分析、测量及处置流程来实现有效的批次效应处理。评测批次效应在单细胞数据分析中极易被忽略,但却有助于判断批次效应的来源、对数据变异的解释度、对数据分析结果的影响度及处理方法,是有效处理批次效应的基础。因此,本篇综述聚焦单细胞转录组数据的批次效应,分别论述批次效应的概念、与普通转录组批次效应的区别、评测方法及面临的挑战,并对未来发展做出展望。 |
关键词: 单细胞测序 批次效应 评测 未来展望 |
DOI:10.12113/202209002 |
分类号:Q2 |
文献标识码:A |
基金项目: |
|
Advancement of research measuring batch effects of single cell RNA transcriptome data |
LI Xiaogang1,2, GAO Zheng1,2, CHEN Jiafeng1,2, FENG Shanru1,2, FU Xiutao1,2, DING Zhenbin1,2,3
|
(1.Department of Liver Surgery & Transplantation, Liver Cancer Institute, Zhongshan Hospital, Fudan University, Shanghai 200032, China; 2.Key Laboratory of Carcinogenesis and Cancer Invasion, Chinese Ministry of Education, Shanghai 200032, China; 3.Shanghai Xuhui Central Hospital, Zhongshan-Xuhui Hospital, Fudan University, Shanghai 200032, China)
|
Abstract: |
Single cell RNA sequencing has emerged as a transformative technology to characterize complex tissues at unprecedented high resolution and answered questions that could not be addressed using traditional bulk RNA sequencing. However, the high complexity and huge data volume of single cell RNA sequencing data presents novel challenges to data analysis, one of which is how to deal with batch effects. Batch effects are technical biases that may cofound results of analyses of high throughput biological data. Meanwhile, the sources of batch effect are complex and the mitigation of batch effect is highly context-dependent. Different processing pipelines including evaluation, analysis, measurement and mitigation are performed, depending on tissue type, sequencing technology, experimental design and so on. The need to measure batch effects is more prone to be neglected in analyses of single cell data. However, measuring batch effects can help identify the source of the batch effects, their proportion of data variation explained and their impact on data analysis and the choice of methods of mitigation, enabling effective handling of the batch effects. Therefore, this review is primarily concerned with batch effects in single cell RNA data, addressing the concepts of batch effects, differences between the handlings of batch effects in single cell RNA data and bulk RNA data, methods of measuring batch effects and major challenges at present. Finally, the future advancement in batch effects measurement is discussed. |
Key words: Single cell sequencing Batch effects Measurement Future advancement |
|
|
|
|