引用本文: | 凌家梅,胡林,陈雪,杨蕊萍,胡迎春.小儿脓毒症休克相关基因的筛选及网络构建[J].生物信息学,2019,17(1):61-66. |
| LING Jiamei,HU Lin,CHEN Xue,YANG Ruiping,HU Yingchun.Gene expression signature analysis and interaction netconstruction of septic shock in children[J].Chinese Journal of Bioinformatics,2019,17(1):61-66. |
|
|
|
本文已被:浏览 2029次 下载 1354次 |
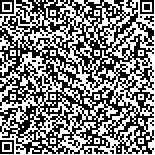 码上扫一扫! |
|
小儿脓毒症休克相关基因的筛选及网络构建 |
凌家梅1,胡林2,陈雪4,杨蕊萍4,胡迎春3
|
(1. 四川省雅安市芦山县人民医院妇产科,四川 雅安 625600;2. 四川省雅安市芦山县人民医院儿科,四川 雅安 625600;3. 西南医科大学附属医院急诊科,四川 泸州 646000;4.西南医科大学临床医学院,四川 泸州 646000)
|
|
摘要: |
为探究脓毒症休克与SIRS的差异表达基因及网络的构建,筛选潜在的核心基因,从GEO数据库下载相关基因表达谱GSE26378,数据分为脓毒症休克与SIRS各29个样本,通过在线软件GCBI对其进行标准化及差异基因筛选;对差异基因进行GO分析;基于KEGG进行功能通路分析以及基因信号网络分析;差异基因共表达网络分析。结果表明: 两组中总共有1 456个基因被识别为差异基因(P<0.05),与SIRS组相比,脓毒症休克组中有条859条下调基因,597条上调基因。GO功能富集分析显示差异基因主要参与了细胞周期、细胞免疫、细胞代谢。KEGG功能通路分析显示差异基因主要参与了MAPK信号通路、P53信号通路、wnt信号通路、细胞凋亡信号通路,细胞周期受体信号通路等。共表达分析发现基因CCNB1 、NUSAP1、OIP5、SHCBP1、ZWINT、TOP2A、DLGAP5等位于网络中央部位,而基因信号网络分析发现基因PLCB1、PIK3CA、STAT3、CAMK2D、PRKCB、CREB1位于网络核心。基因芯片分析有助于发现脓毒症休克与SIRS患儿外周血单核细胞在转录组学上的改变,而生物信息学网络分析有助于发现潜在的靶点。 |
关键词: 脓毒症休克 SIRS 生物信息学 基因信号网络 基因共表达网络 |
DOI:10.12113/j.issn.1672-5565.201805002 |
分类号:Q343.1 |
文献标识码:A |
基金项目: |
|
Gene expression signature analysis and interaction netconstruction of septic shock in children |
LING Jiamei1, HU Lin2, CHEN Xue4, YANG Ruiping4, HU Yingchun3
|
(1.Department of Obstetrics and Gynecology, Lushan County Peoples Hospital, Yaan City, Sichuan Province, Yaan 625600, Sichuan China;2. Department of Paediatrics, Lushan County Peoples Hospital, Yaan City, Sichuan Province, Yaan 625600,Sichuan China;3.Emergency Department of Affiliated Hospital of Southwest Medical University,Luzhou 646000,Sichuan China;4. College of Clinical Medical,Southwest Medical University, Luzhou 646000,Sichuan,China)
|
Abstract: |
To explore the differentially expressed genes (DEGs) and the construction networks of septic shock and SIRS and to screen potential coregenes, relevant gene expression profiles of GSE26378 were downloaded from the GEO database. The data can be divided into 29 septic shock samples and 29 SIRS samples. By the online software GCBI, standardization and differential gene screening, differential gene GO analysis, KEGG functional pathway analysis, gene signal network analysis, and differential gene co-expression network analysis were carried out. Results showed that a total of 1 456 genes in the two groups were identified as DEGs (P<0.05). Compared with the SIRS group, there were 859 down-regulated genes and 597 up-regulated genes in septic shock group. GO enrichment analysis showed that the DEGs were mainly involved in cell cycle, cell immunity, and cell metabolism. KEGG functional pathway analysis showed that the DEGs were mainly involved in MAPK signaling pathway, P53 signaling pathway, Wnt signaling pathway, apoptosis signaling pathway, cell cycle receptor signaling pathway, and other signal pathways. Co-expression analysis showed that CCNB1, NUSAP1, OIP5, SHCBP1, ZWINT, TOP2A,and DLGAP5were located in the central part of the network. Gene signal network analysis showed that PLCB1, PIK3CA, STAT3, CAMK2D, STAT3,and PIK3CAwere located at the core of the network. Microarray analysis is helpful to detect the transcriptional changes of peripheral blood mononuclear cells in children with septic shock or SIRS, and bioinformatics network analysis helps to identify potential targets. |
Key words: Septic shock SIRS Bioinformatics Gene signal network Gene co expression network |
|
|
|
|